本文介绍Databricks数据洞察产品中Databricks Runtime Delta和社区开源版本Delta Lake在性能优化方面的差异点。
Performance Optimization
1. Compaction
Delta Lake on Databricks can improve the speed of read queries from a table by coalescing small files into larger ones.
Official Document: https://docs.databricks.com/delta/optimizations/file-mgmt.html#compaction-bin-packing
Syntax
OPTIMIZE delta.`/data/events`
Or
OPTIMIZE events
Specify an optional partition predicate
OPTIMIZE events WHERE date >= '2017-01-01'
# Databricks notebook source
# DBTITLE 1,Clean up Parquet tables
# %fs rm -r /tmp/flights_parquet
# DBTITLE 1,Clean up Databricks Delta tables
# %fs rm -r /tmp/flights_delta
# DBTITLE 1,Step 0: Read flights data
flights = spark.read.format("csv") \
.option("header", "true") \
.option("inferSchema", "true") \
.load("/databricks-datasets/asa/airlines/2008.csv")
# DBTITLE 1,Step 1: Write a Parquet based table using flights data
flights.write.format("parquet").mode("overwrite").partitionBy("Origin").save("/tmp/flights_parquet")
# Once step 1 completes, the "flights" table contains details of US flights for a year.
# Next in Step 2, we run a query that get top 20 cities with the highest monthly total flights on the first day of week.
# DBTITLE 1,Step 2: Run a query
from pyspark.sql.functions import count
flights_parquet = spark.read.format("parquet").load("/tmp/flights_parquet")
display(flights_parquet.filter("DayOfWeek = 1").groupBy("Month","Origin").agg(count("*").alias("TotalFlights")).orderBy("TotalFlights", ascending=False).limit(20))
# Once step 2 completes, you can observe the latency with the standard "flights_parquet" table.
# In step 3 and step 4, we do the same with a Databricks Delta table. This time, before running the query, we run the `OPTIMIZE` command to ensure data is optimized for faster retrieval.
# DBTITLE 1,Step 3: Write a Databricks Delta based table using flights data
flights.write.format("delta").mode("overwrite").partitionBy("Origin").save("/tmp/flights_delta")
# DBTITLE 1,Step 3 Continued: OPTIMIZE the Databricks Delta table
display(spark.sql("DROP TABLE IF EXISTS flights"))
display(spark.sql("CREATE TABLE flights USING DELTA LOCATION '/tmp/flights_delta'"))
display(spark.sql("OPTIMIZE flights"))
# DBTITLE 1,Step 4 : Rerun the query from Step 2 and observe the latency
flights_delta = spark.read.format("delta").load("/tmp/flights_delta")
display(flights_delta.filter("DayOfWeek = 1").groupBy("Month","Origin").agg(count("*").alias("TotalFlights")).orderBy("TotalFlights", ascending=False).limit(20))
# The query over the Databricks Delta table runs much faster after `OPTIMIZE` is run.
# How much faster the query runs can depend on the configuration of the cluster you are running on,
# however should be **15-20X faster**compared to the standard parquet table.
Benchmark
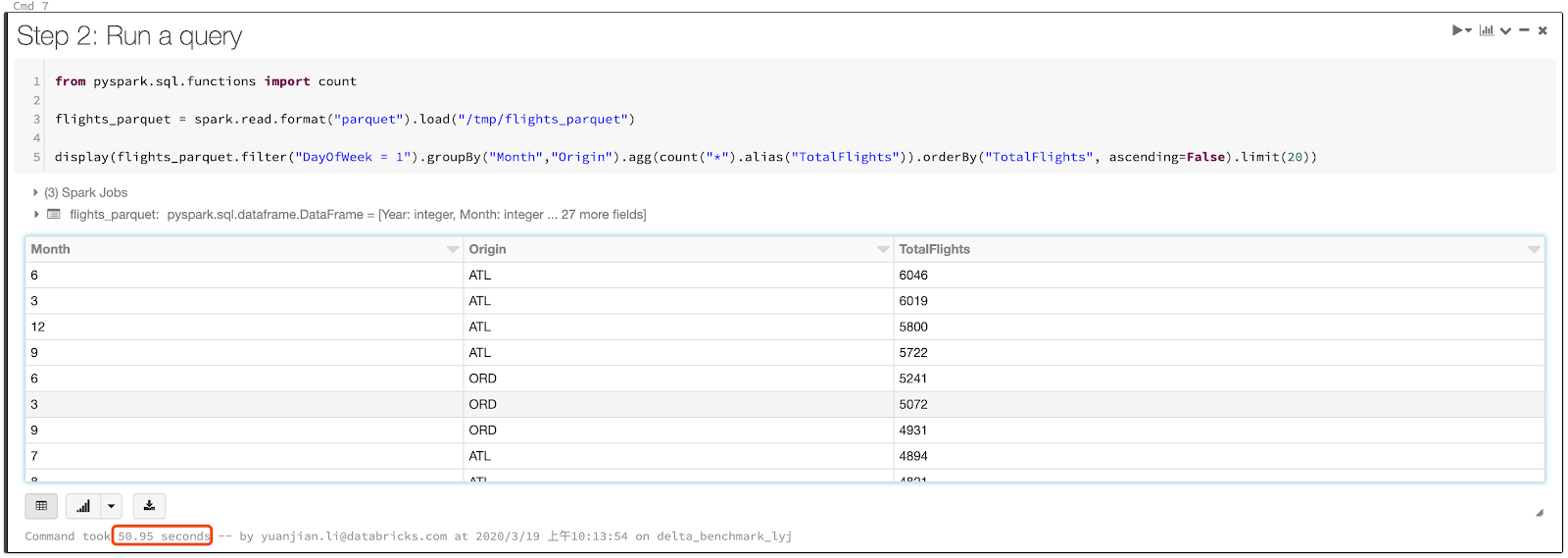
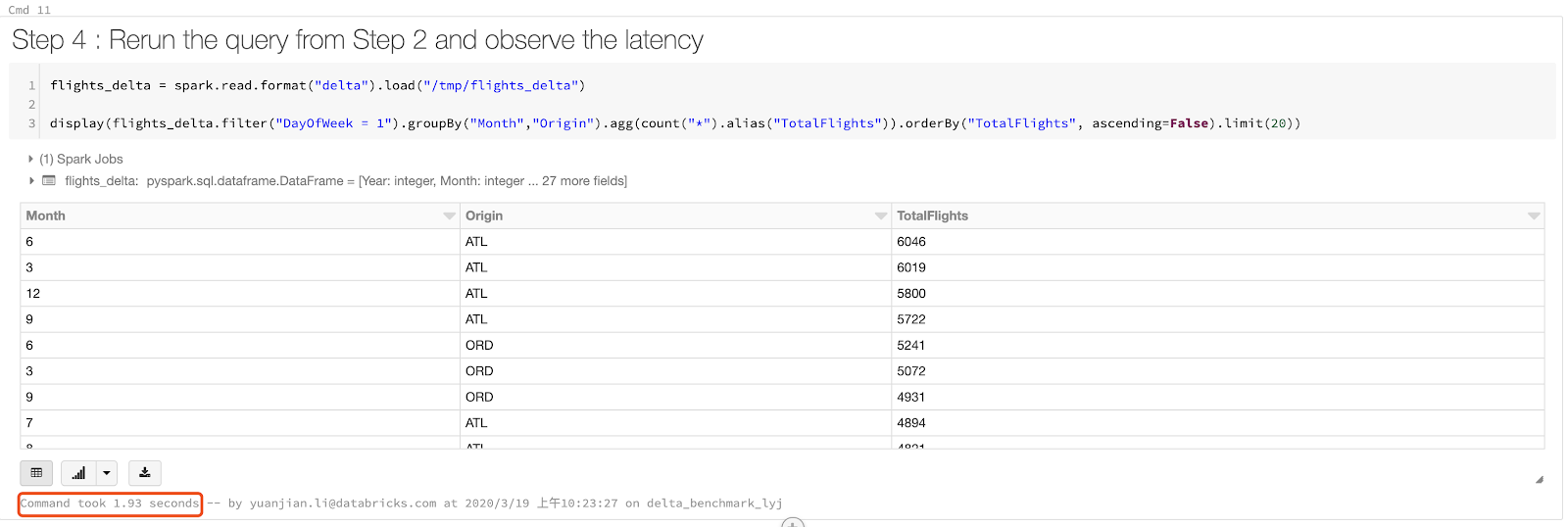
Test Environment | Query over Parquet Table | Query over Delta Table after `OPTIMIZE` is run |
---|---|---|
![]() |
50.95 seconds | 1.93 seconds |
2. Data Skipping
Data skipping information is collected automatically when you write data into a Delta table. Delta Lake on Databricks takes advantage of this information (minimum and maximum values) at query time to provide faster queries. You do not need to configure data skipping - the feature is activated whenever applicable.
Official Document: https://docs.databricks.com/delta/optimizations/file-mgmt.html#compaction-bin-packing
Blog and User Cases: https://databricks.com/blog/2018/07/31/processing-petabytes-of-data-in-seconds-with-databricks-delta.html?_ga=2.29295480.552083878.1584501563-968665100.1584501563
3. Z-Ordering
Z-Ordering is a technique
Syntax
OPTIMIZE events
WHERE date >= current_timestamp() - INTERVAL 1 day
ZORDER BY (eventType)
Demo
All the script for data generation and test code can be found
in: https://drive.google.com/drive/u/1/folders/1ZQKoku9zFoC4qwYY3jlKlhYlvF8I2SpI
-- Dataset schema:
CREATE TABLE `conn_zorder` (`src_ip` STRING, `src_port`INT, `dst_ip` STRING, `dst_port` INT)
USING delta
OPTIONS (
`serialization.format`
'1',
path 'hdfs://rhodium-tests-1:8020/tmp/data/random/conn_zorder')
-- Optimize command:
OPTIMIZE '${baseLocation + connZorder}' ZORDER BY(src_ip, src_port, dst_ip, dst_port)
-- Query Tested:
select count(*) from conn_zorder where src_ip like '157%'and dst_ip like '216.%'
Benchmark
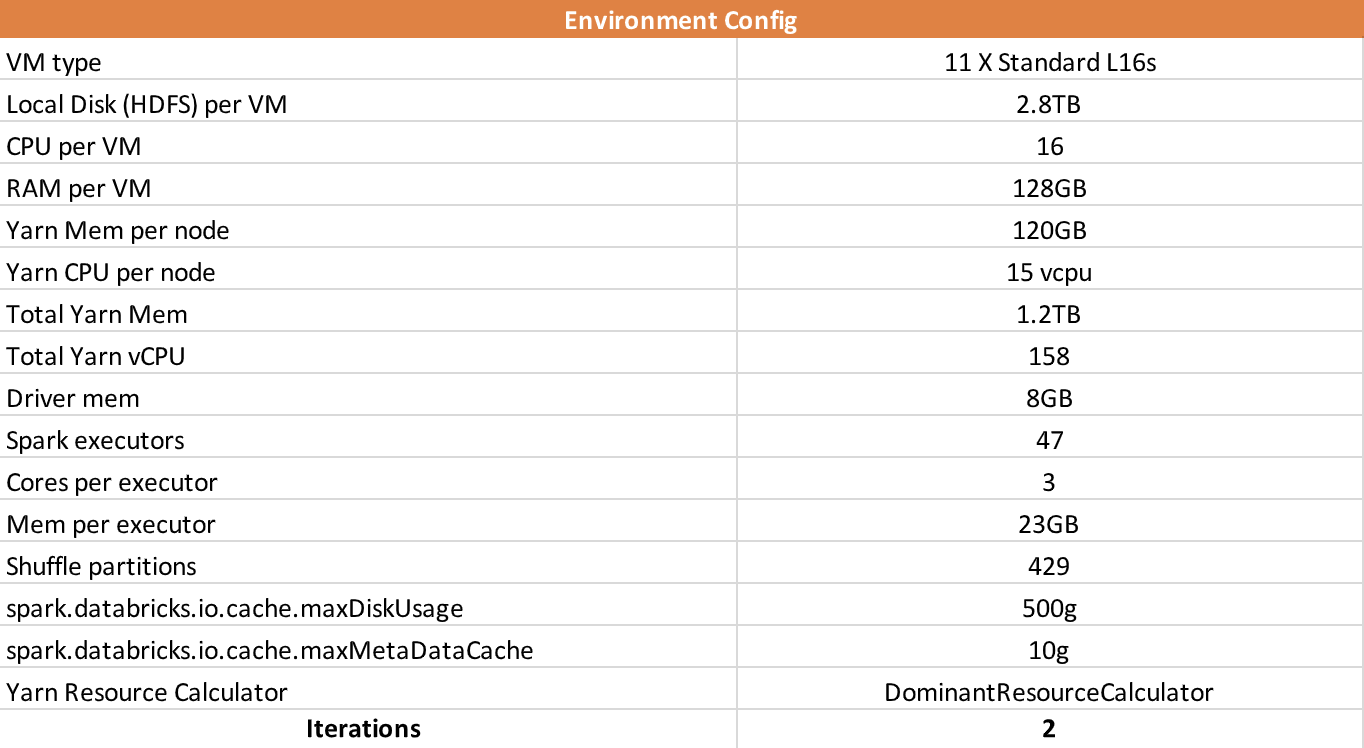
Product | Data Set Size | Runtime of Predicate Query on optimized Columns (s) | DBR Delta faster than OSS Parquet by factor of |
---|---|---|---|
DBR Delta | 10B Row, 1M files (267GB) | 11 | 16.9090909 |
OSS Parquet | 10B Row, 1M files (267GB) | 186 |