本文介绍如何使用AnalyticDB PostgreSQL向量数据库构建文本语义检索系统。
背景信息
文本语义检索旨在通过理解查询文本的意图以及文本含义来得到更好的搜索结果。和传统的词法搜索不同,词法搜索通常只关注查询的关键词,而不理解查询的整体含义。因此,文本语义检索在提高检索性能方面具有巨大的潜力。
文本语义检索概述
文本语义检索的架构如下图所示,通常包括两个组件:
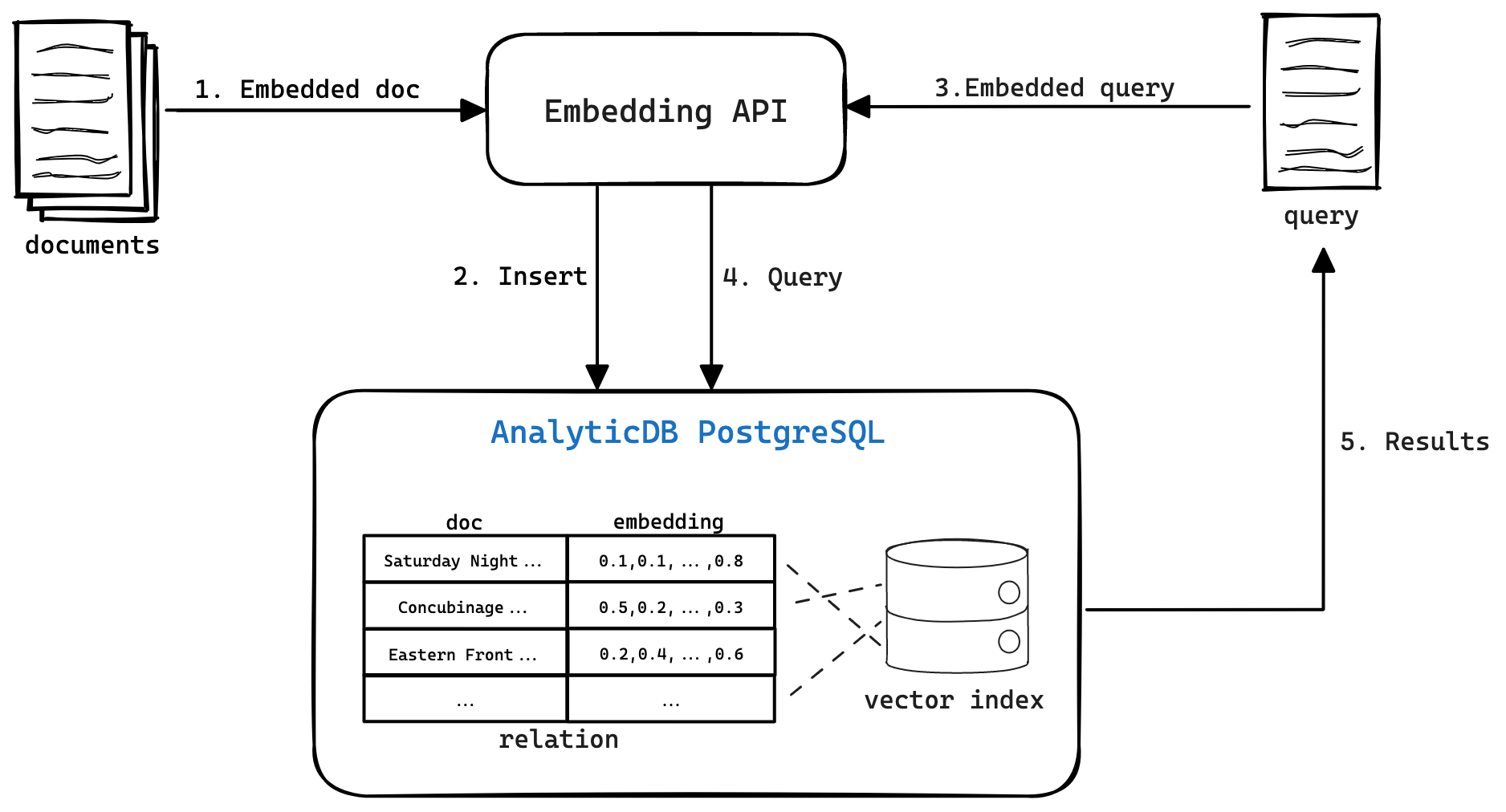
文本向量化和索引构建
文本向量化:指利用机器学习模型将文本转换为向量特征。机器学习模型能够对文本进行编码,使得在语义上与其他文本相似的文本,被编码为在向量空间上彼此接近的向量。您可以使用阿里云提供的NLP基础功能,或者其他文本向量化服务(如OpenAI API提供的Embedding服务)实现文本向量化。
索引构建:将文本及其向量保存到AnalyticDB PostgreSQL版向量数据库中,并为这些向量构建向量索引。向量索引能够极大加速相似向量的查询。
向量检索
查询文本向量化:将查询文本输入到机器学习模型中,得到对应的向量表示。
将得到的向量作为查询向量输入到AnalyticDB PostgreSQL版向量数据库中进行查询。
AnalyticDB PostgreSQL版向量数据库根据向量相似度,从数据库中获取最相似的文档,返回给用户。
使用AnalyticDB PostgreSQL向量数据库构建文本语义检索系统
基于AnalyticDB PostgreSQL版向量数据库构建文本语义检索系统,主要包含以下步骤:
步骤一:安装Python环境。
步骤二:数据预处理。
步骤三:构建文档库及向量索引。
步骤四:查询。
安装Python环境
推荐您使用Conda来管理不同版本的Python环境。本文为测试建立一个新的Python虚拟环境,并安装所需要的库。您可以在终端执行以下命令进行安装。
# 创建新的Python虚拟环境,指定Python版本为python3.8。
conda create -n adbpg_text_env python=3.8
# 激活Python虚拟环境。
conda activate adbpg_text_env
# 在虚拟环境中安装必要的Python包。
pip install psycopg2==2.9.3
pip install wget==3.2
pip install pandas==1.2.4
pip install datasets==2.12.0 sentence-transformers==2.2.2
pip install torch==2.0.1 torchvision==0.15.2 torchaudio==2.0.2
如果您使用的是MAC OS系统,在安装psycopg2时如遇到报错Error: pg_config executable not found.
,可以按照如下方法操作。
先安装postgresql。
brew install postgresql
再安装psycopg2。
pip install psycopg2==2.9.3
数据预处理
为了构建文本语义检索系统,首先需要选择一批文本作为文档库,本文以Quora数据集为例,将Quora数据集中的问题作为文档库为您展示如何利用AnalyticDB PostgreSQL版向量数据库构建文本语义检索系统。
下载数据集
首先利用Huagging Face提供的Datasets包下载Quora数据集。Quora数据集包含有约400000条数据,本文仅使用了其中的10000条数据。
from datasets import load_dataset
dataset = load_dataset('quora', split='train[0:10000]')
print(dataset[0])
每条数据内容如下:
questions包含了2个来自于Quora中的问题。
id对应两个问题的编号。
text为问题文本。
is_duplicate指出这两个问题是否具有相同的含义。
True:两个问题具有相同的含义。
False:两个问题具有不同的含义。
{'questions':
{'id': [1, 2],
'text': ['What is the step by step guide to invest in share market in india?', 'What is the step by step guide to invest in share market?']
},
'is_duplicate': False
}
提取文本对应的向量特征
下载好数据集后,将其中的问题提取出来得到一个文本列表。由于数据集的样本中可能会包含重复的问题,因此我们利用集合过滤掉重复的文本。
sentences = [] for data in dataset['questions']: sentences.extend(data['text']) # 去除重复的文本。 sentences = list(set(sentences)) print('\n'.join(sentences[1:5])) print(len(sentences))
返回结果如下。
How can I know if my spouse is cheating? Can a snake kill a rabbit? How i get hair on bald head? How can I get my name off the first page on Google search? 19413
利用SentenceTransformer库提取文本的向量特征,本文使用了经典的all-MiniLM-L6-v2模型。
from sentence_transformers import SentenceTransformer import torch model = SentenceTransformer('all-MiniLM-L6-v2', device='cpu') model
all-MiniLM-L6-v2模型的重要信息如下所示。
max_seq_length
表示模型能够处理的最大文本长度为256,超出这个长度的文本将会被截断。word_embedding_dimension
表示模型输出的向量特征维度。SentenceTransformer( (0): Transformer({'max_seq_length': 256, 'do_lower_case': False}) with Transformer model: BertModel (1): Pooling({'word_embedding_dimension': 384, 'pooling_mode_cls_token': False, 'pooling_mode_mean_tokens': True, 'pooling_mode_max_tokens': False, 'pooling_mode_mean_sqrt_len_tokens': False}) (2): Normalize() )
依次提取每个文本的向量特征,并将文本及向量特征保存到CSV文件中。该CSV文件包含三列,分别为:
ID:文本编号。
Sentences:文本。
Vectors:文本对应的向量特征。
import pandas as pd vectors = [] for sentence in sentences: vector = model.encode(sentence) # 将向量特征处理成'{0.04067191854119301, ..., -0.012967484071850777}'的格式,方便导入到AnalyticDB PostgreSQL向量数据库中。 vector_str = "{" + ", ".join(str(x) for x in vector.tolist()) + "}" vectors.append(vector_str) # 生成ID列。 ids = [i + 1 for i in range(len(sentences))] # 将ID、Sentences和Vectors合并成一个DataFrame。 df = pd.DataFrame({'ID': ids, 'Sentences': sentences, 'Vectors': vectors}) df.to_csv('sentences_vectors.csv', index=False)
构建文档库及向量索引
设置临时环境变量,并连接到数据库,然后执行一个简单的命令检测是否连接成功。
import os import psycopg2 # 参照以下代码设置临时环境变量。 # os.environ["PGHOST"] = "xxx.xxx.xxx.xxx" # os.environ["PGPORT"] = "58894" # os.environ["PGDATABASE"] = "postgres" # os.environ["PGUSER"] = "vector_test" # os.environ["PGPASSWORD"] = "password" connection = psycopg2.connect( host=os.environ.get("PGHOST", "localhost"), port=os.environ.get("PGPORT", "5432"), database=os.environ.get("PGDATABASE", "postgres"), user=os.environ.get("PGUSER", "user"), password=os.environ.get("PGPASSWORD", "password") ) cursor = connection.cursor() # 执行一个简单的查询测试连接是否正常。 cursor.execute("SELECT 1;") result = cursor.fetchone() if result == (1,): print("Connection successful!") else: print("Connection failed.")
返回
Connection successful!
表明成功连接数据库。创建用于保存文本以及向量特征的表,并在vector上创建向量索引。
说明创建索引时,参数
dim
需要和all-MiniLM-L6-v2模型输出的向量特征维度保持一致,即384。# 用于创建表的SQL,并修改向量列的存储格式为PLAIN。 create_table_sql = ''' CREATE TABLE IF NOT EXISTS public.articles ( id INTEGER NOT NULL, sentence TEXT, vector REAL[], PRIMARY KEY(id) ) DISTRIBUTED BY(id); ALTER TABLE public.articles ALTER COLUMN vector SET STORAGE PLAIN; ''' # 用于创建向量索引的SQL。 create_indexes_sql = ''' CREATE INDEX ON public.articles USING ann (vector) WITH (dim = '384', hnsw_m = '100', pq_enable='0'); ''' # 执行上述SQL。 cursor.execute(create_table_sql) cursor.execute(create_indexes_sql) connection.commit()
将sentences_vectors.csv中处理好的数据导入到表中。
import io # 定义一个生成器函数,逐行处理文件中的数据。 def process_file(file_path): with open(file_path, 'r') as file: for line in file: yield line # 导入数据的SQL。 copy_command = ''' COPY public.articles (id, sentence, vector) FROM STDIN WITH (FORMAT CSV, HEADER true, DELIMITER ','); ''' # 执行COPY命令。 modified_lines = io.StringIO(''.join(list(process_file('sentences_vectors.csv')))) cursor.copy_expert(copy_command, modified_lines) connection.commit()
查询
在完成数据导入后,对于给定的查询,您可以利用AnalyticDB PostgreSQL版向量数据库快速从文档库中检索到在语义上和查询文本最相似的文本。查询通常包括以下步骤:
获取查询对应的向量表示。
利用向量执行引擎从文档库中查询到与查询向量最相似(最相近)的文本。
示例
随机选取一条数据作为查询文本。本文以给定的查询文本“What courses must be taken along with CA course?”为例,AnalyticDB PostgreSQL版向量数据库能够高效准确地找出最相关的文本。
def query_analyticdb(collection_name, query, query_embedding, top_k=20):
# 向量索引列。
vector_col="vector"
# 创建查询SQL。
query_sql = f"""
SELECT id, sentence, dp_distance({vector_col},Array{query_embedding}::real[]) AS similarity
FROM {collection_name}
ORDER BY {vector_col} <-> Array{query_embedding}::real[]
LIMIT {top_k};
"""
# 执行查询。
connection = psycopg2.connect(
host=os.environ.get("PGHOST", "localhost"),
port=os.environ.get("PGPORT", "5432"),
database=os.environ.get("PGDATABASE", "postgres"),
user=os.environ.get("PGUSER", "user"),
password=os.environ.get("PGPASSWORD", "password")
)
cursor = connection.cursor()
cursor.execute(query_sql)
results = cursor.fetchall()
connection.close()
return results
# 查询文本。
query = "What courses must be taken along with CA course?"
# 获取查询文本对应的向量特征。
query_vector=model.encode(query)
print('query: {}'.format(query))
query_results = query_analyticdb('articles', query, query_vector.tolist(), 10)
for i, result in enumerate(query_results):
print(f"{i + 1}. {result[1]} (Score: {round(result[2], 2)})")
返回结果如下。
query: What courses must be taken along with CA course?
1. What courses must be taken along with CA course? (Score: 1.0)
2. What is the best combination of courses I can take up along with CA to enhance my career? (Score: 0.81)
3. Is it possible to do CA after 12th Science? (Score: 0.66)
4. What are common required and elective courses in philosophy? (Score: 0.56)
5. What are common required and elective courses in agriculture? (Score: 0.56)
6. Which course is better in NICMAR? (Score: 0.53)
7. Suggest me some free online courses that provides certificates? (Score: 0.52)
8. I have only 2 months for my CA CPT exams how do I prepare? (Score: 0.51)
9. I want to crack CA CPT in 2 months. How should I study? (Score: 0.5)
10. How one should know that he/she completely prepare for CA final exam? (Score: 0.48)
您可以继续选择其他文本进行查询。完成后,您可以删除文档库及索引以节省资源。
# 删除文档库的SQL。
drop_table_sql = '''drop table public.articles;'''
cursor.execute(drop_table_sql)
connection.commit()
connection.close()
- 本页导读 (1)